% pip3 install --upgrade openai
openai.ChatCompletion.create() -> client.chat.completions.create()
response = openai.chat.completions.create(
model = model,
messages = messages,
temperature = 0,
)
return response.choices[0].message.content
我实际没有修改client,也是成功的。但是从下面吴恩达的介绍看,response也要修改为属性调用,而不是直接调用。
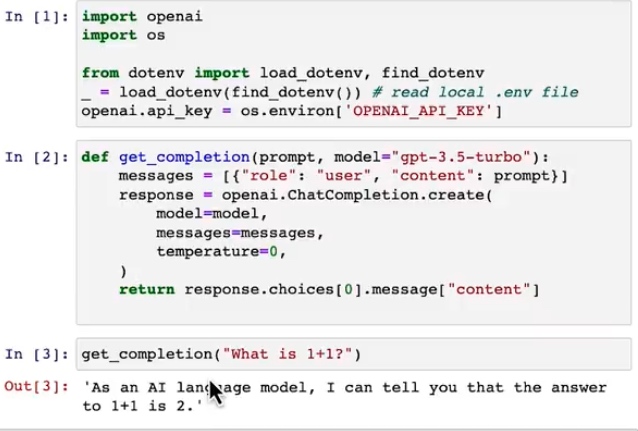
不过经过chatgpt的解释,我还是使用了client这个实例来调用相关函数,原因如下(更灵活,更好支持异步操作、更符合行业惯例)
The shift from using openai.chat to client.chat in the OpenAI API reflects a change in the design of the OpenAI Python library. Here are the key reasons for this change:
Client Instance Configuration:
Using a client instance allows for more flexible configuration. You can create multiple client instances with different configurations (e.g., different API keys, different base URLs for testing or production environments) within the same application. This is more scalable and adaptable for complex applications or those that need to interact with multiple environments or configurations.
Asynchronous Support:
The new API design is likely structured to better support asynchronous operations. By using a client object, it's easier to manage asynchronous requests and responses, which are becoming increasingly important for efficient handling of network operations, especially in web applications and services.
Consistency with Other SDKs:
Many modern API SDKs (Software Development Kits) use a similar pattern where you create a client instance and then use methods on this instance to interact with the API. This design pattern is familiar to many developers and aligns with best practices in software development.
Encapsulation and Extensibility:
By using a client object, the OpenAI library can encapsulate functionality and state more effectively. This makes the library easier to extend and maintain. It also allows for better handling of resources like network connections.
Error Handling and Debugging:
A client-based approach can offer improved error handling and debugging capabilities. Since each client instance can manage its own state and configuration, it's easier to trace and handle errors, log activities, and debug issues.
In summary, the shift to using a client instance in the OpenAI Python library is likely driven by a desire for greater flexibility, support for asynchronous operations, consistency with modern software development practices, improved encapsulation and extensibility, and enhanced error handling and debugging capabilities.
更详细的异步调用的好处
The emphasis on asynchronous support in the new API design, particularly with the use of a client object, reflects a broader trend in software development towards more efficient and scalable network operations. Here's a deeper look into why this is beneficial:
1. Improved Performance and Scalability:
Non-blocking Calls: Asynchronous operations allow your application to make non-blocking network calls. This means that your application doesn't have to wait for a response from the OpenAI server before continuing with other tasks. This is especially beneficial in web applications where multiple users might be making requests at the same time.
Handling Multiple Requests: Asynchronous programming is more efficient at handling multiple simultaneous network requests. This is crucial for high-load applications that need to maintain responsiveness under heavy request volumes.
2. Better Resource Utilization:
Concurrency: Asynchronous operations enable better utilization of system resources. While waiting for a response from an API call, your application can perform other tasks, thereby making better use of the CPU and other resources.
Reduced Latency: In a synchronous model, each operation must complete before the next one starts, potentially leading to higher latency. Asynchronous operations can overlap, which can reduce overall latency in the application.
3. Enhanced User Experience:
Responsive Applications: In a web or mobile application, asynchronous operations can significantly enhance the user experience. Users aren't left waiting for operations to complete and can continue interacting with other parts of the application.
Real-time Updates: Asynchronous programming facilitates real-time updates to the user interface, which can be crucial for applications that require immediate feedback, such as chatbots or live data monitoring.
4. Simplified Error Handling:
Asynchronous workflows: Asynchronous programming often comes with more sophisticated ways to handle errors and exceptions. For instance, in Python's asyncio, you can use try/except blocks within asynchronous functions to manage exceptions effectively.
5. Alignment with Modern Web Standards:
WebSockets and HTTP/2: Modern web protocols like WebSockets and HTTP/2 are designed to work efficiently with asynchronous communication, making it a natural fit for applications that leverage these technologies.
Implementation in Python:
asyncio Library: Python’s asyncio library is a popular choice for writing asynchronous code. It provides a framework for writing single-threaded concurrent code using coroutines, multiplexing I/O access, and running network clients and servers.
Integration with Frameworks: Many modern Python web frameworks (like FastAPI, Sanic, etc.) are built with native support for asynchronous operations, making it easier to integrate with asynchronous APIs like OpenAI's.
In summary, the shift towards asynchronous support with a client object in API design is a response to the growing need for more efficient, scalable, and responsive applications, particularly in the context of web and network services. It aligns well with modern software practices and technological advancements.
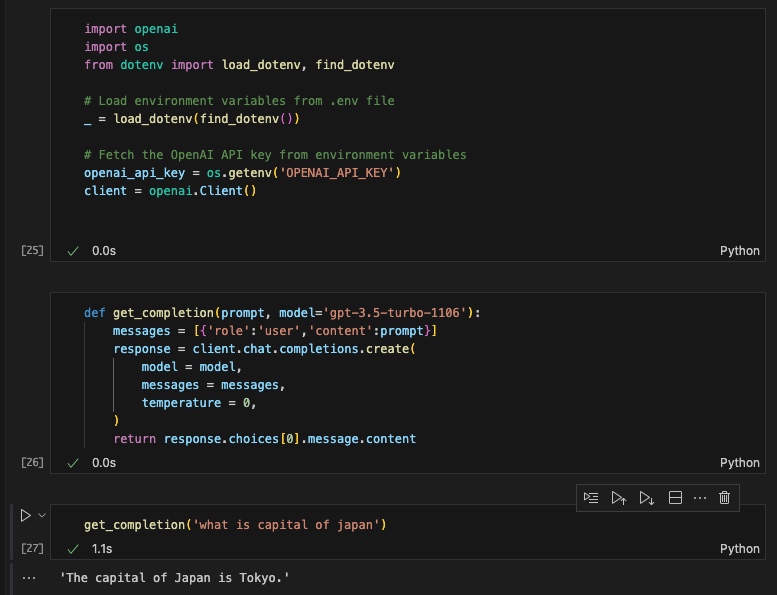
参考这篇文档